The Values in Viral Dispersion (VIVID) Project has two aims. The first is to make available practically useful computational simulations of human factors in managing the COVID-19 pandemic, such as compliance with physical distancing, social networks, and trust in public health officials. The second aim is to educate policy professionals and the public about computational simulation, helping them become more sophisticated consumers of information derived from pandemic-related models.
Testing policy options in a simulated artificial environment provides an ethical way to conduct experiments that generate valuable insights into likely policy outcomes. As the artificial society is refined and expanded, it can be modified to test policy solutions for various aspects of social life that have been altered in this pandemic. Our simulation is also being used to study restarting and maintaining the educational sector, from K-12 schools to colleges and universities.
Many groups have policy questions and they need guidance urgently. Should staff with pre-existing conditions that render them vulnerable to COVID-19 be segregated? In schools, should class schedules be rearranged to reduce the number of kids and teachers in school at a time? Do more people wear masks in public when local government is policing it?
Questions such as these can be evaluated in artificial worlds, measuring policy combinations against the criteria that matter most to policymakers, leaders, and experts. Is financial survival the main priority? Minimizing infections? Protecting vulnerable people? Equity across different communities? One-size-fits-all advice won’t work because it doesn’t take account of the human values that people use to figure out what counts as success for them in their specific context.
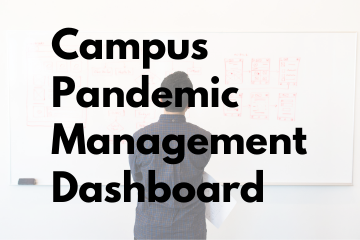
Approach
Modeling & simulation can be used to reveal the internal causal dynamics of the social systems through which the virus spreads, to show policy makers how policies interact and change outcomes, and to inspire new policies through a deeper understanding of the underlying architecture of our value-infused social systems. Our enhanced epidemiological models incorporate crucially important human factors that are often overlooked. Proposing scheduling changes to get a school restarted doesn’t have the same outcome when students are non-compliant. Our models enable us to experiment safely and ethically with policy alternatives, measuring their effectiveness against the precise concerns that stakeholders tell us matter to them. Such insights can never replace local expert judgment, but they can function as vital adjuncts to reasoning about policy consequences.
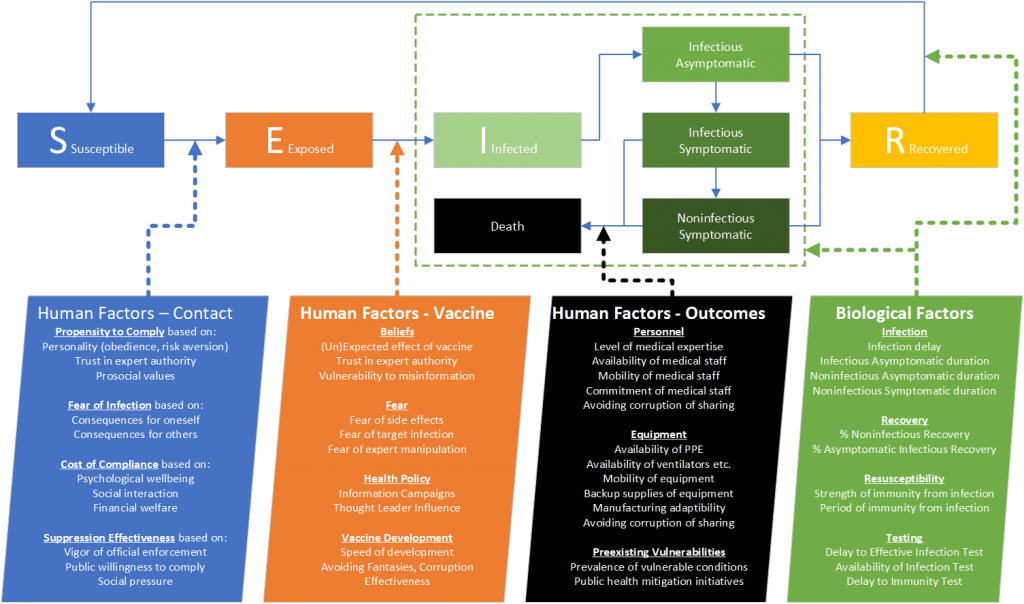
Our multidisciplinary team studies the human factors in complex social systems, approaching the problem from various fields, including the humanities, social science, natural sciences, medical science, and computational sciences. This knowledge sets up the foundation for two SEIRS-based system dynamics models that are eventually merged for a more sensitive prediction of exposure and infection rates due to COVID-19.
Model 1: Top-Down
Model 1 is a system-level model analyzing the dynamics of state changes as a population moves through the SEIRS stages. For example, state government in New Mexico sees positive effects of NPIs in Utah and decides to adopt the same policies and enforcement measures. However, Utah is largely Mormon and folks there tend to comply when religious authorities support NPI rules. New Mexico is more diverse and less compliant, which interferes with the effectiveness of NPIs. This model factors in diverse human beliefs and values that matter in viral spread, such as lack of trust in experts, wariness of vaccines, pro-sociality, and ideological polarization.
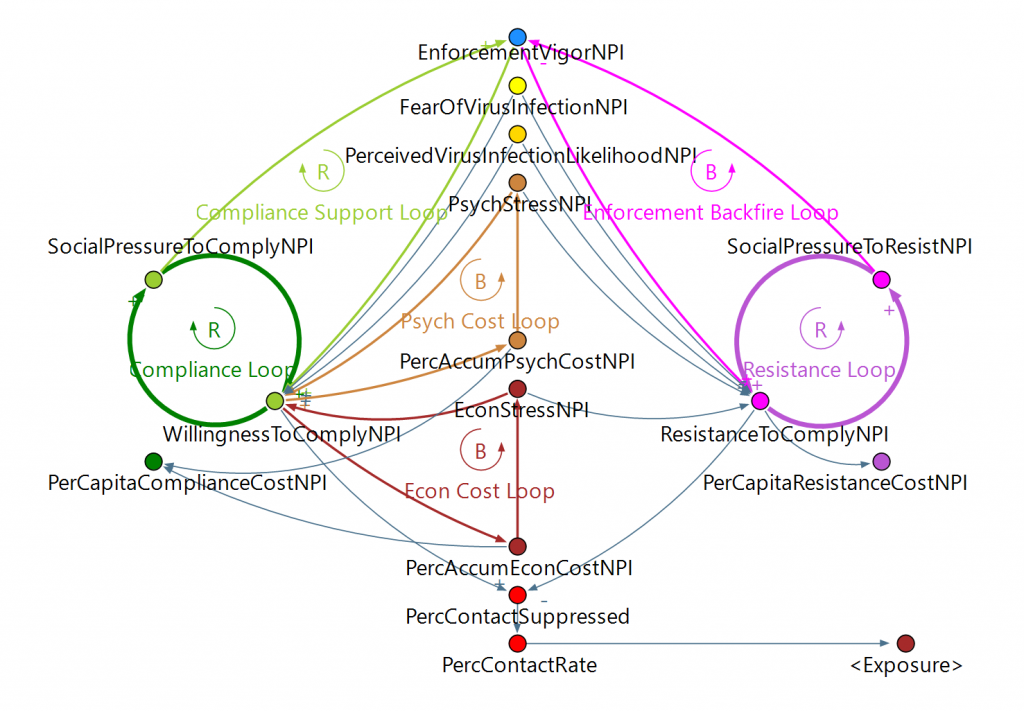
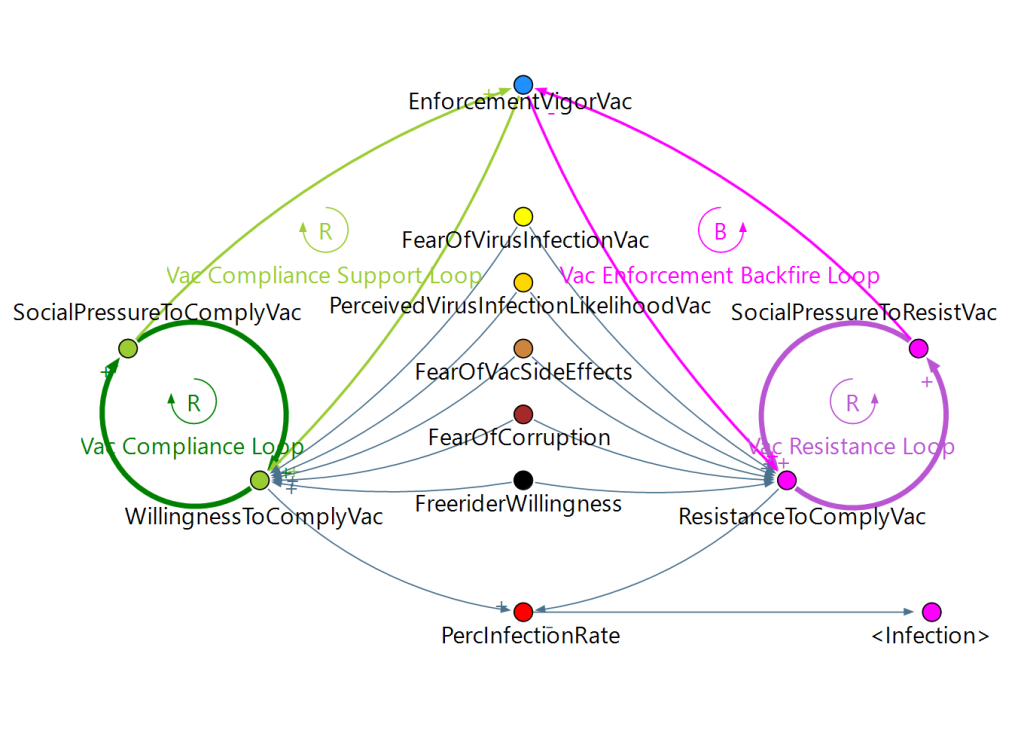
Model 2: Bottom-Up
Model 2 is a social network model that consists of psychologically realistic and behaviorally plausible AI agents that embody human beliefs and values. Real-world communities are mapped onto social networks, which feed into the model. The history of individual agents, contact possibilities using varied social networks, and real-world distributions of resources all impact the outcome. The model explores dynamics of disease diffusion in several types of networks.
Based on distance – people are linked if the distance between them is less than a given value.
Random – a person is linked to a random subset of the population.
Small World – most links are neighbors, but there is a certain percent of long-range links.
Scale Free – some people are “hubs” with lots of connections and some are “hermits”.
Ring Lattice – each person is linked to a fixed number of his closest neighbors on a ring.
Custom Networks – reflecting real-world social networks such as schools.
The agent-based model becomes a platform for virtual experimentation with policy options. It’s sensitive to both diverse metrics and values that define individuals and systems and to distinctive movement, scheduling, and social-network realities.
Impact
This project aims to develop a more sensitive prediction of exposure and infection rates due to COVID-19 by accounting for the impact of human values on viral spread, the social consequences of the pandemic, and people’s varying attitudes toward vaccines. Policy proposals can be tested in a simulated environment in a way that attaches measures to outcomes and optimizes against key criteria. Concerns that stakeholders, policymakers, and leaders have about policy proposals can be applied to the specific situation within the models. Model 1 has more generic applications and is based on situation type. The findings are faster, yet coarser. Model 2 has precise applications and is based on situation details. The findings are finer, yet slower. With the merging of both models, findings are more holistic and the policy proposals can be better evaluated to inform decisions.
Publications
Andrew Page, Saikou Y. Diallo, Wesley J. Wildman, George Hodulik, Eric W. Weisel, Neha Gondal, David Voas. “Computational Simulation Is a Vital Resource for Navigating the COVID-19 Pandemic,” Simulation in Healthcare: The Journal of the Society for Simulation in Healthcare. February 2022.
Rich Byrne, Gail H. Cassell, Matthew E. Downs, Florida International University, John Halamka, Shayri M. Kansagra, Leavitt Partners, Rakhee Palekar, Neelima Ramaraju, Kunal J. Rambhia, Kippy Rudy, Jay Schnitzer, Taylor Wilkerson. “Planning for On-Campus K-12 Education During COVID-19,” COVID-19 Healthcare Coalition. August 6, 2020.
Recent updates
External Resources:
“COVID-19 Decision Support Dashboard,” COVID-19 Healthcare Coalition: https://c19hcc.org/resources/decision-support/
“The Health and Economic Impacts of COVID-19 Interventions,” RAND Corporation: https://www.rand.org/pubs/tools/TLA173-1/tool.html
“Modeling Innovation,” Virginia Modeling, Analysis and Simulation Center: https://medium.com/modeling-innovation